The role storage plays in the AI data cycle
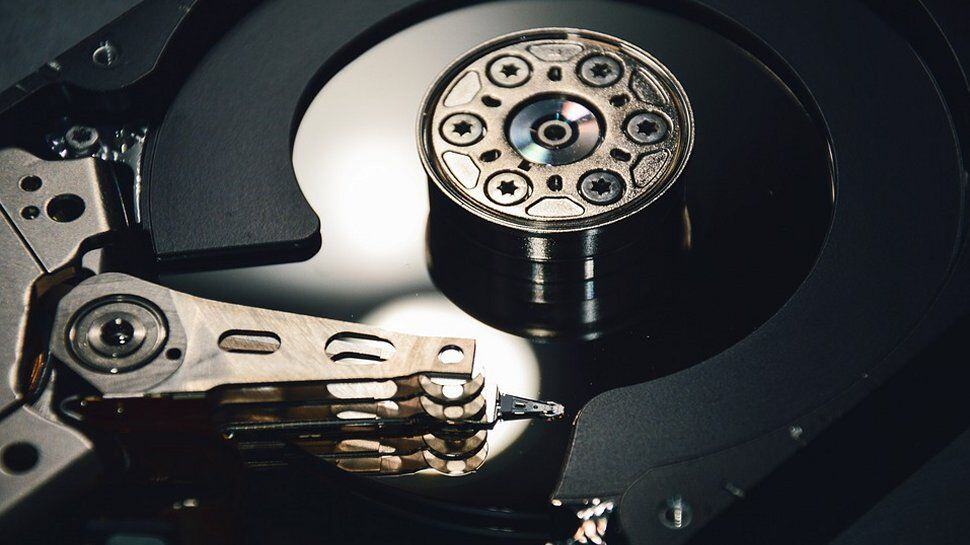

As the Artificial Intelligence (AI) industry continues to mature, it necessitates the development of robust infrastructure to train models and deliver services – greatly impacting data storage and management. This has significant implications for the amount of data generated and most importantly, how and where to store this insight.
The ability to manage this data efficiently is becoming critical as data requirements increase exponentially due to the continuous growth and development of AI tools. Therefore, the storage infrastructure needed to support these systems must be able to scale in parallel with the rapid advancements in AI applications and capabilities.
With AI creating new data and making existing data even more valuable, a cycle quickly emerges, where increased data generation leads to expanded storage needs. This fuels further data generation – forming a “virtuous AI data cycle” which drives AI development forward. To fully leverage AI’s potential, organizations must not only grasp this cycle, but fully understand its implications for infrastructure and resource management.
Peter Hayles, Product Marketing Manager HDD, Western Digital.
A six stage AI data cycle
The AI Data Cycle consists of a six-stage framework designed to streamline data handling and storage. The first stage is focused on collecting existing raw data and storage. Data here is collected and stored from various sources, and the analysis of the quality and diversity of collected data is critical – setting the base for the next stages. For this stage of the cycle, capacity enterprise hard disk drives (eHDDs) are recommended, as they deliver the highest capacity per drive and lowest cost per bit.
The next stage is where data is prepared for intake and the evaluation from the previous stage is administered, prepared and transformed for training purposes. To accommodate this stage, datacentres are applying upgraded storage infrastructure – like fast data lakes – to support data for preparation and intake. Here, high-capacity SSDs are needed to enhance existing HDD storage or to create new all-flash storage systems. This ensures swift access to organised and prepared data.
Then comes the next phase of training of AI models to make accurate projections with training data. This phase typically occurs on high-performance supercomputers – requiring specific and high-performance storage solutions to operate as effectively as possible. Here, high-bandwidth flash storage and low-latency enhanced eSSDs are created to meet the specific needs of this stage, providing necessary speed and precision.
Next, following training, the inference and prompting stage focuses on the creation of a user-friendly interface for AI models. This stage incorporates the use of an application programming interface (API), dashboards and tools that combine context to specific data with end-user prompts. Then, AI models will integrate into internet and client applications without needing to interchange current systems. This means that maintaining current systems alongside new AI computing will require further storage.
Sign up to the TechRadar Pro newsletter to get all the top news, opinion, features and guidance your business needs to succeed!
Here, larger and faster SSDs are essential for AI upgrades in computers, and higher-capacity embedded flash devices are needed for smartphones and IoT systems to maintain seamless functionality in real-world applications.
The AI inference engine stage follows, where trained models are positioned into production environments to perform the examination of new data, produce new content or provide real-time predictions. At this stage, the engine’s level of efficiency is critical in achieving quick and accurate AI responses. Therefore, to ensure a comprehensive data analysis, significant storage performance is essential. To support this stage, high-capacity SSDs can be used for streaming or to model data into inference servers based on scale or response time needs, while high-performance SSDs can be used for caching.
The final stage is where the new content is created, with insights produced by AI models and then stored. This stage completes the data cycle, by continually enhancing data value for future model training and analysis. The generated content will be stored away on enterprise hard drives for datacenter archive purposes and in both high-capacity SSDs and embedded flash devices for AI edge devices, making it readily available for future analysis.
A self-sustaining data generation cycle
By fully understanding the six stages of the AI data cycle and employing the right storage tools to support each phase, businesses can effectively sustain AI technology, streamline their internal operations, and maximize the benefits of their AI investment.
Today’s AI applications use data to produce text, video, images and various other forms of interesting content. This continuous loop of data consumption and generation accelerates the need for performance-driven and scalable storage technologies for managing large AI datasets and re-factoring complex data efficiently, driving further innovation.
The demand for appropriate storage solutions will significantly increase in time as the role of AI across operations becomes even more prevalent and integral. As a result, the access to data, the efficiency and accuracy of AI models, and larger, higher-quality datasets will also become increasingly important. Additionally, as AI becomes embedded across nearly every industry, partners and customers can expect to see storage component providers tailor their products so that there is an appropriate solution at each and every stage of the AI data cycle.
We’ve featured the best data recovery service.
This article was produced as part of TechRadarPro’s Expert Insights channel where we feature the best and brightest minds in the technology industry today. The views expressed here are those of the author and are not necessarily those of TechRadarPro or Future plc. If you are interested in contributing find out more here: https://www.techradar.com/news/submit-your-story-to-techradar-pro
As the Artificial Intelligence (AI) industry continues to mature, it necessitates the development of robust infrastructure to train models and deliver services – greatly impacting data storage and management. This has significant implications for the amount of data generated and most importantly, how and where to store this insight. The…
Recent Posts
- Everything new on Disney+ in March 2025: Marvel’s Daredevil: Born Again, Moana 2, Sadie Sink’s O’Dessa movie, and more
- The best Apple Watch in 2025
- Volvo ES90 will charge faster, drive farther than other Volvo EVs
- The truth about GenAI security: your business can’t afford to “wait and see”
- H&R Block Coupons and Deals: 20% Off Tax Prep in 2025
Archives
- February 2025
- January 2025
- December 2024
- November 2024
- October 2024
- September 2024
- August 2024
- July 2024
- June 2024
- May 2024
- April 2024
- March 2024
- February 2024
- January 2024
- December 2023
- November 2023
- October 2023
- September 2023
- August 2023
- July 2023
- June 2023
- May 2023
- April 2023
- March 2023
- February 2023
- January 2023
- December 2022
- November 2022
- October 2022
- September 2022
- August 2022
- July 2022
- June 2022
- May 2022
- April 2022
- March 2022
- February 2022
- January 2022
- December 2021
- November 2021
- October 2021
- September 2021
- August 2021
- July 2021
- June 2021
- May 2021
- April 2021
- March 2021
- February 2021
- January 2021
- December 2020
- November 2020
- October 2020
- September 2020
- August 2020
- July 2020
- June 2020
- May 2020
- April 2020
- March 2020
- February 2020
- January 2020
- December 2019
- November 2019
- September 2018
- October 2017
- December 2011
- August 2010