One step closer to the Matrix: AI defeats human champion in Street Fighter — with a revolutionary type of memory it used makes it even more powerful

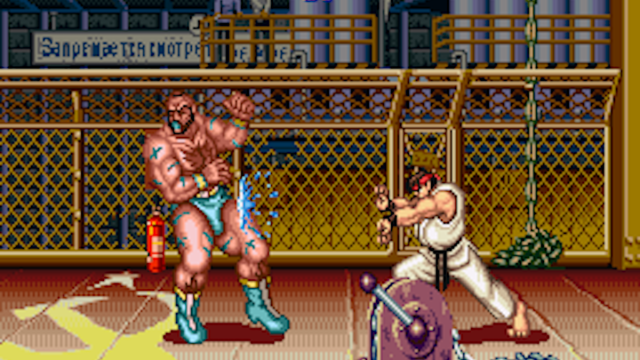
Researchers from the Singapore University of Technology and Design (SUTD) created a new software centered around reinforcement learning and phase-change memory that’s designed to understand complicated movement design.
Previous work has applied this kind of deep learning to other games like Chess or Go, but they decided instead to expose the D-PPO algorithm to the rigors of Street Fighter Champion Edition II. The SUTD researchers trained its SF-R2 AI player on two days of consecutive play against the computer, before letting it loose on a human participant – who the AI-powered system beat comfortably.
The work has implications for movement science more broadly, according to the research paper, and can possibly be fed into improving robotics and autonomous vehicles, for example. It paves the way for broadly applicable training in fields where machines may observe human norms and attempt to replicate and outperform them.
Ready Pl-AI-yer One
One of the major milestones that AI researchers have used to measure the effectiveness of the systems they’ve built is by letting them compete with human players in different kinds of games. This has been happening for some time.
In 2017, an Alpha Go AI built by DeepMind beat the number-one human Go player in the world for the second time, following the first victory over Fan Hui the previous year. Microsoft’s AI, in June, achieved the world’s first perfect Ms. Pac-Man score, and in August we saw an OpenAI engine beating the best Dota 2 players of the time.
This latest milestone – besting a Street Fighter champion – was made possible due to reinforcement learning as well as phase-change memory. First developed by HP, this is a form of nonvolatile memory achieved by using electrical charges to change areas on chalcogenide glass. It’s much faster than commonly used Flash memory.
“Our approach is unique because we use reinforcement learning to solve the problem of creating movements that outperform those of top human players,” said principal investigator Desmond Loke to TechXplore. “This was simply not possible using prior approaches, and it has the potential to transform the types of moves we can create.
More from TechRadar Pro
Researchers from the Singapore University of Technology and Design (SUTD) created a new software centered around reinforcement learning and phase-change memory that’s designed to understand complicated movement design. Previous work has applied this kind of deep learning to other games like Chess or Go, but they decided instead to expose…
Recent Posts
- Die in the Dungeon will keep you busy until Slay the Spire 2
- Sana Grain Mill Review: Makes Specialty Flours a Piece of Cake
- I tested an ultra-cheap Dolby Atmos soundbar against a premium alternative, here’s why it’s worth spending the extra cash
- ‘Revolutionary’ Wi-Fi router which can send data up to 10 miles away goes on sale for less than $100 – just make sure you’re happy with the 32Mbps speed
- The Humane Ai Pin Will Become E-Waste Next Week
Archives
- February 2025
- January 2025
- December 2024
- November 2024
- October 2024
- September 2024
- August 2024
- July 2024
- June 2024
- May 2024
- April 2024
- March 2024
- February 2024
- January 2024
- December 2023
- November 2023
- October 2023
- September 2023
- August 2023
- July 2023
- June 2023
- May 2023
- April 2023
- March 2023
- February 2023
- January 2023
- December 2022
- November 2022
- October 2022
- September 2022
- August 2022
- July 2022
- June 2022
- May 2022
- April 2022
- March 2022
- February 2022
- January 2022
- December 2021
- November 2021
- October 2021
- September 2021
- August 2021
- July 2021
- June 2021
- May 2021
- April 2021
- March 2021
- February 2021
- January 2021
- December 2020
- November 2020
- October 2020
- September 2020
- August 2020
- July 2020
- June 2020
- May 2020
- April 2020
- March 2020
- February 2020
- January 2020
- December 2019
- November 2019
- September 2018
- October 2017
- December 2011
- August 2010