Most formidable supercomputer ever is warming up for ChatGPT 5 — thousands of ‘old’ AMD GPU accelerators crunched 1-trillion parameter models

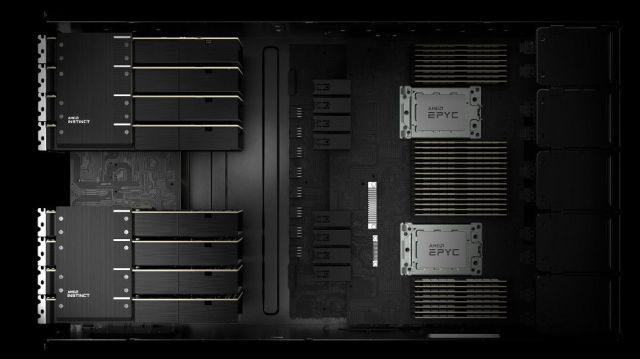
The most powerful supercomputer in the world has used just over 8% of the GPUs it’s fitted with to train a large language model (LLM) containing one trillion parameters – comparable to OpenAI‘s GPT-4.
Frontier, based in the Oak Ridge National Laboratory, used 3,072 of its AMD Radeon Instinct GPUs to train an AI system at the trillion-parameter scale, and it used 1,024 of these GPUs (roughly 2.5%) to train a 175-billion parameter model, essentially the same size as ChatGPT.
The researchers needed 14TB RAM minimum to achieve these results, according to their paper, but each MI250X GPU only had 64GB VRAM, meaning the researchers had to group up several GPUs together. This introduced another challenge in the form of parallelism, however, meaning the components had to communicate much better and more effectively as the overall size of the resources used to train the LLM increased.
Putting the world’s most powerful supercomputer to work
LLMs aren’t typically trained on supercomputers, rather they’re trained in specialized servers and require many more GPUs. ChatGPT, for example, was trained on more than 20,000 GPUs, according to TrendForce. But the researchers wanted to show whether they could train a supercomputer much quicker and more effectively way by harnessing various techniques made possible by the supercomputer architecture.
The scientists used a combination of tensor parallelism – groups of GPUs sharing the parts of the same tensor – as well as pipeline parallelism – groups of GPUs hosting neighboring components. They also employed data parallelism to consume a large number of tokens simultaneously and a larger amount of computing resources. The overall effect was to achieve a much faster time.
For the 22-billion parameter model, they achieved peak throughput of 38.38% (73.5 TFLOPS), 36.14% (69.2 TFLOPS) for the 175-billion parameter model, and 31.96% peak throughput (61.2 TFLOPS) for the 1-trillion parameter model.
They also achieved 100% weak scaling efficiency%, as well as an 89.93% strong scaling performance for the 175-billion model, and an 87.05% strong scaling performance for the 1-trillion parameter model.
Although the researchers were open about the computing resources used, and the techniques involved, they neglected to mention the timescales involved in training an LLM in this way.
TechRadar Pro asked the researchers for timings, but they have not responded at the time of writing.
More from TechRadar Pro
The most powerful supercomputer in the world has used just over 8% of the GPUs it’s fitted with to train a large language model (LLM) containing one trillion parameters – comparable to OpenAI‘s GPT-4. Frontier, based in the Oak Ridge National Laboratory, used 3,072 of its AMD Radeon Instinct GPUs…
Recent Posts
- Elon Musk’s AI said he and Trump deserve the death penalty
- The GSA is shutting down its EV chargers, calling them ‘not mission critical’
- Lenovo is going all out with yet another funky laptop design: this time, it’s a business notebook with a foldable OLED screen
- Elon Musk’s first month of destroying America will cost us decades
- The first iOS 18.4 developer beta is here, with support for Priority Notifications
Archives
- February 2025
- January 2025
- December 2024
- November 2024
- October 2024
- September 2024
- August 2024
- July 2024
- June 2024
- May 2024
- April 2024
- March 2024
- February 2024
- January 2024
- December 2023
- November 2023
- October 2023
- September 2023
- August 2023
- July 2023
- June 2023
- May 2023
- April 2023
- March 2023
- February 2023
- January 2023
- December 2022
- November 2022
- October 2022
- September 2022
- August 2022
- July 2022
- June 2022
- May 2022
- April 2022
- March 2022
- February 2022
- January 2022
- December 2021
- November 2021
- October 2021
- September 2021
- August 2021
- July 2021
- June 2021
- May 2021
- April 2021
- March 2021
- February 2021
- January 2021
- December 2020
- November 2020
- October 2020
- September 2020
- August 2020
- July 2020
- June 2020
- May 2020
- April 2020
- March 2020
- February 2020
- January 2020
- December 2019
- November 2019
- September 2018
- October 2017
- December 2011
- August 2010