Machine learning and evolving threats Machine learning and evolving threats

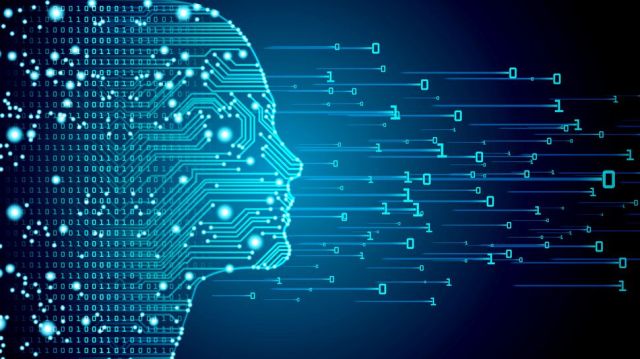
Cybercriminals today are extremely organized and often take advantage of social trends to deliver weaponized bundles used to launch an attack against victims. These bundles are typically delivered via phishing emails or malware web sites that include misinformation targeting fears and uncertainty. This technique often correlates with major trends or events, such as the pandemic, social movements like Black Lives Matter, or important governmental changes, for instance, the upcoming U.S. presidential election.
In recent months, for example, threat intelligence researchers have been seeing an evolution in ransomware attacks targeting those most impacted by COVID-19, such as hospitals and health care providers. In fact, 41 hospitals announced ransomware attacks during the first half of 2020.
Ransomware gangs, typically associated with well-established and known criminal organizations are also evolving their tactics for extortion, including publicly shaming victim organizations and threatening to publish files to the internet or auction off PII (personally identifiable information) to the highest bidder.
Organized cybercrime
Organized cybercrime is nothing new, however. For years, researchers have been following the commercialization of malware, with many criminal groups developing affiliate programs that pay cybercriminals to help spread and evolve a particular strain of malware. One such example is the wildly successful GandCrab ransomware, which allegedly reaped more than U.S. $2 billion in earnings for the group behind the malware in a 15-month period beginning January 2018.
Ransomware-as-a-service is just one example of how malware has been commercialized, enabling cybercriminals to quickly and easily deliver modularized attacks that are easily purchased and pieced together to launch and relaunch an attack with the click of a button. As threat actors continuously evolve and adapt their tactics, techniques, and procedures (TTPs), security researchers are challenged with staying abreast of their behaviors in order to provide timely, accurate threat intelligence that supports effective threat detection and response.
Machine learning can provide solutions for data problems
One of the biggest challenges threat researchers face is the sheer volume of information they must sift through, including collecting, normalizing, validating, and analyzing threat data — all of which are very time-consuming tasks. For example, AT&T Alien Labs™, the threat intelligence unit of AT&T Cybersecurity, ingests as much as 20 million threat artifacts per day. This includes global observations on the evolving TTPs of threat actors, including the common tools, IT infrastructure, and other means they use in attacks. In order to turn this information into curated threat intelligence, threat researches must go through multiple steps of validation and analysis — and they must do this quickly to keep pace with cybercriminals.
Even as researchers struggle to sift through mountains of data, the move to a distributed workforce adds yet another challenge by increasing the size and complexity of the attack surface an organization’s security team is tasked with protecting 24/7/365. Without the context of continuously updated and actionable threat intelligence, SOC analysts end up drowning in alerts. In fact, 63 percent of companies at the lower end of cybersecurity maturity and 52 percent of companies in the middle noted in a recent survey that they ignore more than 25 percent of security events — that’s one-quarter of events that could be hiding a potential adversary somewhere in the network.
To address the big data problem, threat researchers are increasingly turning to machine learning as a way to facilitate threat analysis and essentially help them find the proverbial needle in the haystack. This is especially so considering that 76 percent of security professionals believe they face a cybersecurity skills shortage — meaning, they are already stretched thin in their roles. For example, machine learning can assist researchers by enriching information around indicators of compromise (IoCs) — the traces of evidence that help security professionals to detect an attack. This includes helping to identify and predict infrastructure associated with IoCs, such as command and control (C&C) servers, IP addresses used to launch attacks, or newly registered domains that will eventually be used for malicious purposes.
Machine learning models used to identify, predict, and detect malware
A report published by the Telco Security Alliance (TSA) in July 2020 observed that members of the Alien Labs Open Threat Exchange™ (OTX™) contributed more than 1 million COVID-related IoCs between January and June, 2020. The FBI has also reported similar figures revealing that cybercrime has increased 400 percent since COVID-19 started.
To create more efficiency in identifying and detecting malware during these peak periods of activity, machine learning models can be used to identify and predict the behaviors of malware families as they propagate and morph. For example, models can group malware files into clusters, which helps to speed the identification and classification of current and evolving families. To do this, data scientists utilize a specific dataset of known malware files, and then use it to train algorithms to find patterns and make predictions about new data coming in. These techniques can also provide a better understanding of the constantly evolving TTPs of adversaries.
More than ever, being able to discover, identify, and predict these macro behaviors is essential to providing resiliency in threat detection and response, especially as networks are rapidly morphing to keep up with the fast-changing work environment and threat landscape. Organized cybercrime and state-sponsored groups are showing no signs of slowing — in fact, it has become easier and faster than ever for cybercriminals to launch new campaigns.
All of this leads to one end: more threats, more variations, on threats, and more information and data to sift through. We’ve seen this to be especially true during times of crisis. However, armed with data analytics, automation, and machine learning, threat researchers have the tools needed to stay abreast of adversary TTPs, and to produce one of the most valuable controls in security —- validated, actionable, and continuously updated threat intelligence.
Cybercriminals today are extremely organized and often take advantage of social trends to deliver weaponized bundles used to launch an attack against victims. These bundles are typically delivered via phishing emails or malware web sites that include misinformation targeting fears and uncertainty. This technique often correlates with major trends or…
Recent Posts
- Prime Video puts a Supernatural spin on The Boys season 5 cast as Jared Padalecki and Misha Collins sign on to the popular show in mystery roles
- Elon Musk and DOGE are using Slack, Salesforce CEO Benioff says
- Invincible season 3 episode 6’s mid-credits scene just confirmed the Prime Video show’s next two episodes will be an absolute bloodbath
- Should ransomware payments be illegal?
- Engwe Mapfour N1 Pro e-bike review: the new ‘premium’
Archives
- February 2025
- January 2025
- December 2024
- November 2024
- October 2024
- September 2024
- August 2024
- July 2024
- June 2024
- May 2024
- April 2024
- March 2024
- February 2024
- January 2024
- December 2023
- November 2023
- October 2023
- September 2023
- August 2023
- July 2023
- June 2023
- May 2023
- April 2023
- March 2023
- February 2023
- January 2023
- December 2022
- November 2022
- October 2022
- September 2022
- August 2022
- July 2022
- June 2022
- May 2022
- April 2022
- March 2022
- February 2022
- January 2022
- December 2021
- November 2021
- October 2021
- September 2021
- August 2021
- July 2021
- June 2021
- May 2021
- April 2021
- March 2021
- February 2021
- January 2021
- December 2020
- November 2020
- October 2020
- September 2020
- August 2020
- July 2020
- June 2020
- May 2020
- April 2020
- March 2020
- February 2020
- January 2020
- December 2019
- November 2019
- September 2018
- October 2017
- December 2011
- August 2010